OEE
Changing Consumer Attitudes on Sustainability
Data collection has become a major part of food manufacturing, but without data standardization, measuring overall equipment effectiveness (OEE) poses a challenge.
OEE Metrics Evolve
as Data Standardization Takes Shape
The race to standardize plant data from programmable logic controllers (PLC), sensors and production lines is happening fast. As food manufacturers standardize data, digital tools accelerate and provide a closer look at overall equipment effectiveness (OEE) and maintenance metrics.
Food manufacturing is the largest employer among manufacturing industries, employing more than 1.7 million workers. However, labor shortages in the food segment have been well-documented and currently sit where employment was in 2017 and 2018. With many food plants reporting capacity utilization, any shocks to labor availability will add more challenges to plant managers and corporate general managers.
As shown, the last 10 years have been challenging for hiring and retaining qualified plant workers. Food companies have responded to these challenges by investing in advanced technology and moving toward more automation. Recent surveys point to more capital expenditures for predictive maintenance, with many corporate projects executing data standardization.
In a recent PMMI survey, food industry personnel were asked how they were leveraging technology to remedy any knowledge or skills gap in their operations. Integrating smart technology to assist operations and maintenance took the top spot at 44%. The following two spots were automating through large language models (LLMs) at 38% and standardization at 31%.
Food operations are finding increased throughput and troubleshooting line faults due to new digital tools or middleware technology. In the process, companies are solving bottlenecks faster due to production line and PLC data standardization.
“When you get into multiple plants, companies want manufacturing execution systems (MES) in their food and beverage plants,” says John Oskin, SVP at SmartSights. “So, when you do that, there's absolutely an agenda to standardize data with automation investments.”
Photo courtesy: Getty Images / yoh4nn
By Grant Gerke
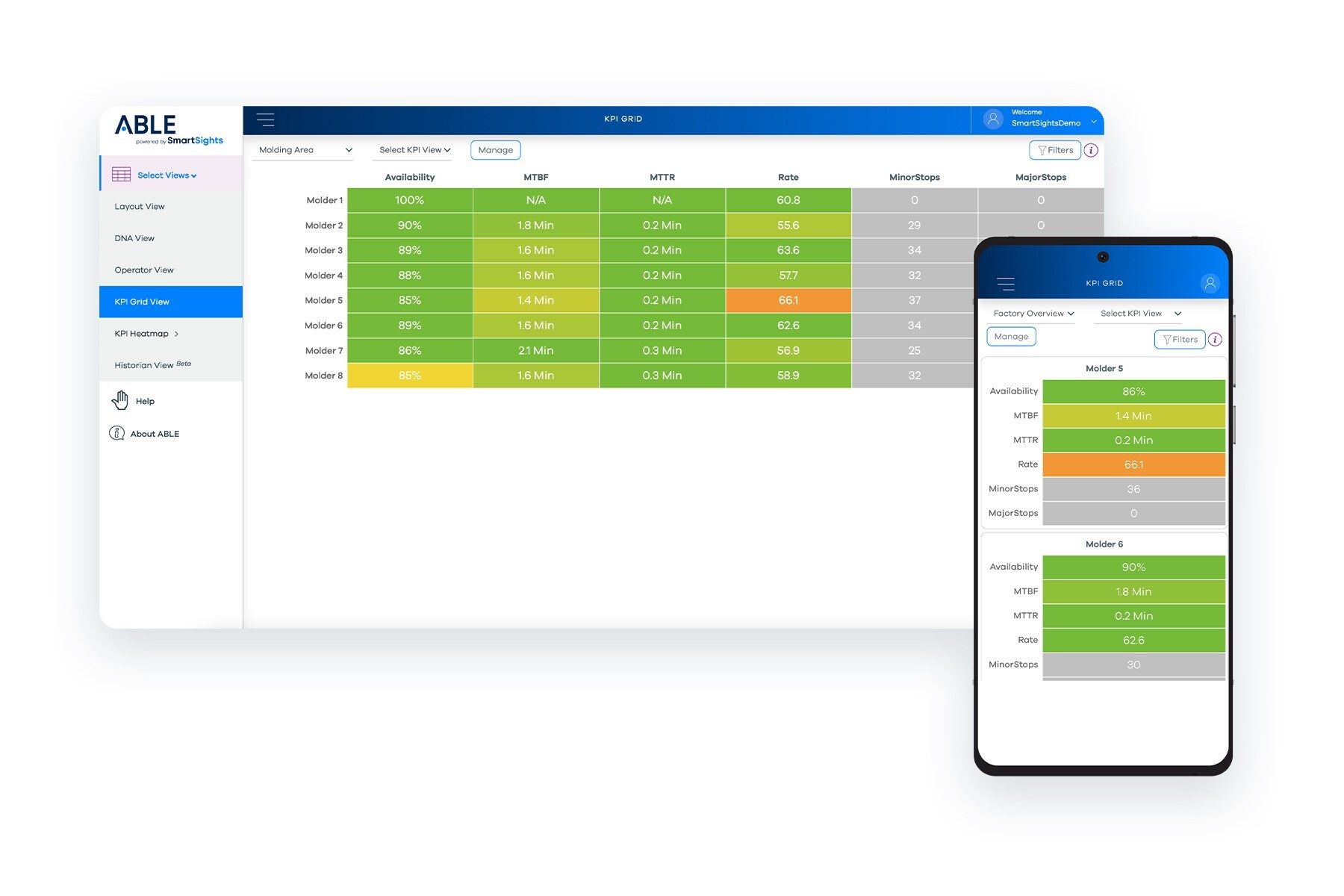
SmartSights’ technology collects machine-level information, which can be five to 10 times richer than line data collection. Source: SmartSights
Standardizing Data for Trusted OEE
Industry 4.0’s promise is to connect plant floor production to middleware and, eventually, to an ERP system. But that’s predicated on clean and standardized data.
“When we started MontBlancAI two-and-a-half years ago, we had this naive view that manufacturers had good data sources since machines are excellent data sources,” says Markus Guerster, founder and CEO of MontBlancAI. “We learned that the data sources are great, but the technology that collects, cleans and stores the machine information is not good at handling significant data volume.” MontBlancAI’s machine-learning AI solution relies on actionable data from PLCs and sensors.
“While there was a focus on maintenance metrics, especially OEE, there was no standardized way to measure across the enterprise,” adds Ravishankar Subramanyan, HiveMQ director of industry solutions manufacturing. “This would result in companies having many versions of the OEE, which is hard to compare and contrast.”
“Ten years ago, OEE systems were monitoring at the bottleneck, and it was a good strategy, but today, that is certainly not adequate for AI,” Oskin says. “It’s difficult to get the root cause when an operator is looking at a filler asset but not secondary assets, so this necessitates the need for advanced data collection.” SmartSights’ technology allows food and beverage manufacturers to leverage data from machine and line PLCs, with no additional programming needed for data acquisition.
“We have an approach that can combine PLC programs and HMI configuration files to identify key pieces of information for MES and OEE applications. This information is then unified and packaged for rapid deployment,” Oskin says. “Getting consistent PLC data collection is achieved using our templates and adopting standards, such as PackML."
With continued mergers and acquisitions of new plants in the food segment, standardizing OEE and other metrics — mean time between failures (MTBF), mean time to repair (MTTR), asset availability and planned maintenance percentage (PMP) — has been a difficult challenge, but large system software and data standardization are helping.
HiveMQ offers a Message Queuing Telemetry Transport (MQTT) platform that connects manufacturing data throughout the company. “One of the strategies for capturing and standardizing OEE, MTBF, throughput, yield and efficiency metrics is creating a Unified Namespace,” Subramanya says. The company pushes a Unified Namespace for sensors, controllers and equipment, which include downtime events, cycles times, energy consumption and defects.
For HiveMQ, constructing these models involves unifying data from multiple sources and creating a data model for downtime:
- Machine or PLC data indicating when downtime starts and ends
- Manual or automated reason codes for the downtime
- Operator and shift information
- Physical location of the machine
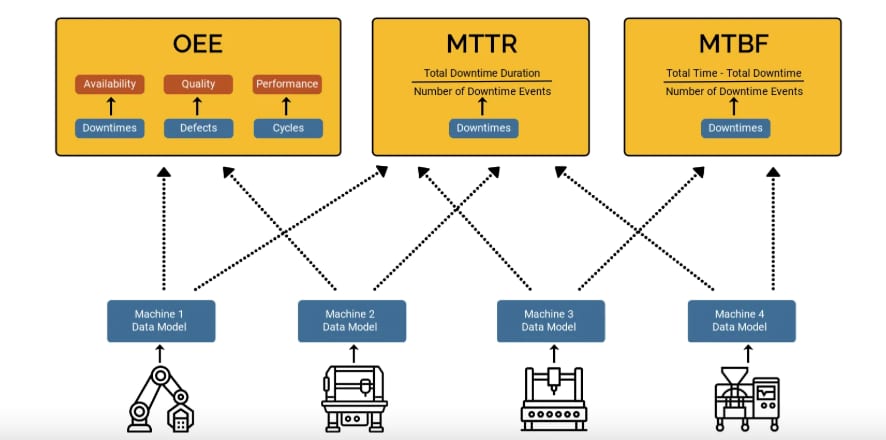
HiveMQ constructs models that involves unifying data from multiple sources and creating a data model for downtime, such as machine or PLC data indicating when downtime starts and ends. Source: HiveMQ
MQTT is being used increasingly as a data protocol with Supervisory Control and Data Acquisition (SCADA) platforms. At a recent Ignition Conference, Shamrock Foods’ Alec Gordon, lead controls engineer, showed how the company collects motor data and uses it to alert maintenance personnel of a potentially failing asset. Shamrock Foods uses Inductive Automation’s Ignition SCADA platform for its plants. With the aid of the SCADA platform, Gordon developed user interfaces for Shamrock Foods Company's maintenance team, including a Variable Frequency Drive (VFD) monitoring screen, a force motor screen to assist with predictive maintenance and a scanner diagnostics screen.
“Our maintenance team was having much trouble diagnosing VFD faults. It was more of a training issue, but we also wanted to provide them with a lot of VFD diagnostic information wherever they ran,” Gordon said during his presentation.
Gordon adds, “So, when we defined our Ignition setup, we used a naming convention for Open Platform Communications (OPC) PLC connections, which defined User Defined Types (UDTs) and employed a multi-instance wizard to define everything uniformly and then applying our historian.” The unified UDTs create tags for each VFD and then are linked to a data historian from Canary Labs.
Canary Labs historians specialize in optimizing and reading time-series data, performing calculations on data sets sent to them and then transferring that data back into Ignition publishing via MQTT. With the use of standardized data tags in the SCADA platform, Shamrock Foods runs calculations on all 382 VFD assets at once, such as percent assets.
“I did it over 45 days since I figured that’d be a good enough snapshot of an asset’s average,” Gordon adds. “If it started to fail for three days, let’s say, you might see some spikes and it could throw off your average, but doing a trend over 45 days should be enough to filter that out and give you a pretty accurate amp.”
More Smart Technology for Maintenance
Various digital tools are emerging for food companies in maintenance and operations. For example, smaller digital twin tool applications are being employed for maintenance to simulate production sequences to provide a more accurate analysis of downtime causes.
“We're capturing uptime, downtime, throughput and reject information accounting for every minute of the day and can synchronize that data across a group of assets on the line or even across lines,” Oskin says.
The digital twin functionality allows a maintenance team to replay and validate the data. “From a plant perspective, many things happen off shift or on third shift, when there’s not a lot of people around,” Oskin adds. “So, the ability to replay this data allows them to see a sequence of downtime that might’ve led to a crash.” FE