Maintenance Strategies
Using Data
Food and beverage manufacturers are discovering that machine learning is a vital ingredient in moving to predictive maintenance strategies. Larger food companies have the jump on digital transformations, but smaller companies are starting small and increasing spending to start the journey.
to Drive Predictive Maintenance Strategies
Doing more with less is a familiar refrain in the maintenance department, but food and beverage manufacturing executives and plant floor personnel are moving toward a full-fledged understanding that capital investments are needed to extend machine lifecycles and production uptime.
What’s driving this realization? There are many, but one is a shared understanding of a limited workforce and that more digital tools are needed. A recent report from Deloitte and the Manufacturing Institute indicates manufacturers may need up to 3.8 million new workers by 2033, with nearly half of these positions potentially going unfilled if current labor gaps persist. Plus, fewer workers are coming into manufacturing, and the current average age of highly skilled workers is 56, with nearly a quarter of this workforce set to retire in the next decade.
The other driver is machine learning (ML) technology, a subset of AI, and a foundational piece of this revolution in manufacturing. For more than 10 years, food manufacturers have added vibration sensors, gathered equipment data and fed information into data analytics platforms while also adding computerized maintenance management systems (CMMS). Food companies are succeeding, but will executives stay committed to larger capital investments in machine learning and analytics, especially when asset management investments are hard to measure?
Workforce Pressures in Maintenance
For the last 10 years, the race has been to move away from reactive maintenance and toward predictive analytical strategies so maintenance teams can schedule fixes during traditional downtime and optimize uptime.
“Predictive maintenance and related technologies have gained more traction in recent years, but there is a long road ahead as most manufacturers still do not understand this new technology and its potential positive impact on their day-to-day operations,” says Ronak Macwan, senior industry marketing manager for manufacturing at Brightly Software, a Siemens company.
“Over the past five years, the roles of maintenance technicians, planners and managers in the food processing segment have shifted significantly,” adds Lucie Dahuron, global marketing cross segment leader at Schneider Electric. “They have become much more proactive than merely reactive in addressing maintenance issues.”
In 2022, grab-and-go sandwich producer E.A. Sween began using Brightly Software’s Asset Essentials CMMS platform that would manage and maintain more than 7,000 parts in the plant.
“We were using a very difficult, paper-driven work order process,” says Erik Willams, maintenance planning and purchasing supervisor at E.A. Sween. “Our purchase orders were paper, and technicians used a sign-out sheet in our parts room to order the parts they needed. It was complicated to track and trace everything.”
The company ramped up production from five to seven days a week in 2022 due to soaring demand, and preventive maintenance completion rates steadily declined to about 25%. With the new CMMS platform, Williams determined that small preventive maintenance jobs had kept the percentage high, while many of the large preventive maintenance tasks remained uncompleted.
“We had these million-dollar pieces of equipment that weren’t getting any preventive maintenance or downtime,” Willams says. “The software showed us that our technicians were just inundated.”
The data also showed that technicians were needed for 120 hours of preventive maintenance during a two-week period, while they were only at work for 80 hours.
“We were able to use that data to show we needed additional preventive maintenance staff, and it gave us the leverage to get those people,” Williams says.
By Grant Gerke
Making a prepared main course in the test kitchen is one thing, but should it be turned into a product offering and, if so, how can the freshness and quality of the components be maintained with problematic suppliers and logistics issues—and if all those things go well, will consumers love your product? AI-based PLM stands ready to help make the right decisions. Image courtesy of Getty Images / yongyuan
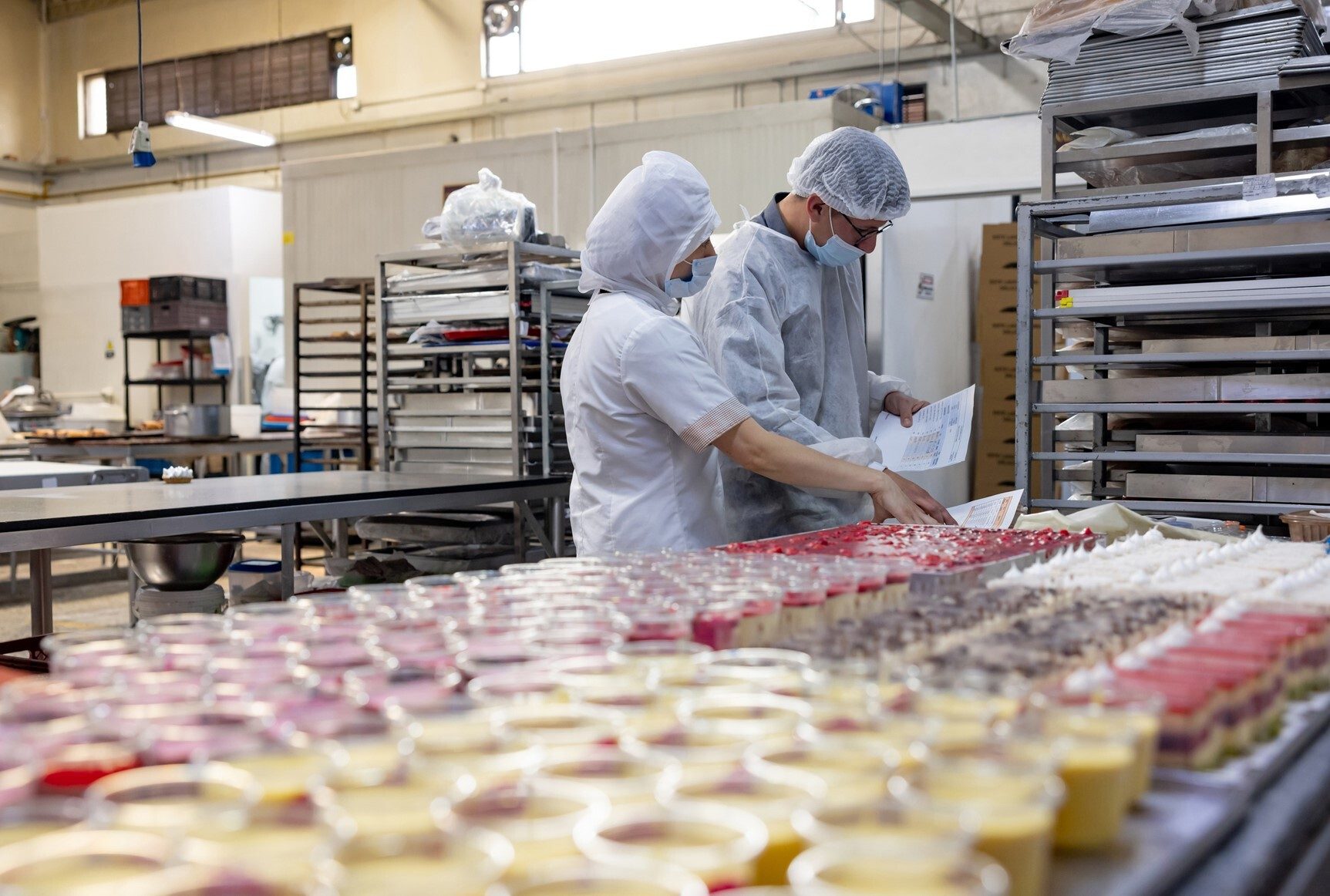
Small and large food manufacturers are adopting digital tools and system software to optimize maintenance while facing workforce challenges. Image courtesy of Brightly Software, a Siemens Company
Brightly Software’s Macwan says data analytics is transforming food and beverage manufacturing’s maintenance processes in ways that mirror E.A. Sween’s experience.
“Historical data plays a significant role in preventive maintenance strategies by providing detailed information on each asset and piece of equipment, such as previous repair history, inventory parts information and current equipment condition,” Macwan says.
The software allows E.A. Sween to set short maintenance windows, automatically assign work, update and quickly reassign when something changes, and the software automatically creates follow-up work orders to keep things moving and maintain equipment.
In addition to flexible maintenance schedules, modern CMMS platforms aid technicians with machine data and images via mobile devices.
“Mobile and augmented reality solutions support the workforce with their daily tasks, and all the information is available on the machine,” Dahuron says. “The software guides the maintenance technician through their tasks and reduces onboarding of new employees.”
Moving to Predictive Maintenance Strategies
While some manufacturers add more digital tools to operations, large food producers and ingredient companies are taking bigger leaps at refining machine data and moving to predictive maintenance strategies. These strategies require a capital expenditure (CAPEX) commitment to data governance and acquisition platforms.
“Food and beverage manufacturers often lack a clear understanding between asset life cycle management and its connection to the capital planning process,” Macwan says. “Food executives must realize that the key to overall success is understanding how to maximize equipment life expectancy and leverage current and historical equipment data for the capital planning process.”
“Data analytics is a perfect way to analyze a huge amount of data and focus only on the 0.1%, which are relevant,” Dahuron adds. “For example, the increase in the feedback time of a valve is a good indicator of the valve’s lifecycle. With the right data, you can select 0.1% of the valves where the feedback time increased most.”
At the recent AVEVA World 2024, Ajinomoto presented its success in moving to a predictive maintenance strategy and increasing uptime and production at its plant in Eddyville, Iowa.
“There were many times where there has been a problem that has suddenly happened where it be a process upset or an equipment failure that then leads to a process upset, just requires immediate attention to alleviate some of those issues,” says Michael Ross, chemical process engineer at Ajinomoto Health and Nutrition North America.
Ajinomoto uses AVEVA’s PI historian for data collection and connects to SAM GUARD software, a machine learning solution that connects, contextualizes and monitors data for anomalies.
“The team is notified of any potential process upsets before they happen, and we are able to then make a decision to alter course and schedule maintenance during a planned downtime period rather than taking unplanned downtime, which is costly,” says Meg Lashier, senior production coordinator at Ajinomoto Health and Nutrition North America.
Ajinomoto moved forward with this approach:
- The first step is to have all historical data points sorted hierarchically within the third-party software.
- Then, it contextualizes the data according to its process flow and assigns different sensors to various assets within the model.
- From there, Ajinomoto trains the model based on two-plus years of process data.
However, bad data can corrupt data modeling and predictive maintenance strategies. “One thing that we had to be very careful of while we were doing this is to take out any sort of process upsets or any major plant shutdowns that we had,” Lashier adds.
With the data modeled, Ajinomoto prioritizes assets and individual sensors by assigning them sensitivity ratings. “A critical asset that we would like to be alerted to and even with small changes outside of what is considered normal data, we will be assigned a very high sensitivity range,” Lashier says. “And operators will be notified more frequently on that asset versus an asset that we know has a range of what is considered normal.”
At the event, Lashier provided an example of its fluidized bed dryer and how the software sent an alert about a set point issue for a motor. The maintenance team troubleshooted but couldn’t find the root cause. “Eventually, we scheduled time to take down the dryer during a normal cleaning day when we had time to do it,” Lashier says. “And ultimately, we found a big mess inside the dryer.” Ajinomoto realized there was caramelized product that was binding and restricting flow, which eventually would’ve backed up and had cascading impacts on the rest of production.
Going forward in 2025, small and large food manufacturers can look for quick wins — as well as large CAPEX investments — to help them move toward a predictive maintenance approach.