Automation
While there are already some AI tools available, proving their effectiveness will take some dedicated teamwork from suppliers, integrators and manufacturers.
Still Needs Fine Tuning To Succeed In Manufacturing
Artificial Intelligence:
When I asked the AI wizard, ChatGPT, how can and how do AI tools make wrong decisions in manufacturing, the AI came up with ten problem areas where manufacturing AI tools need further development and testing to become proficient and accepted. The problem areas listed include (in no particular order): insufficient or inaccurate data, inadequate model training, bias in data, changes in operating conditions, uncertainty and noise, model complexity, lack of context, model drift, human-computer interaction, and adversarial attacks (manipulating inputs to produce incorrect decisions). The trouble is that AI still has a way to go to convince food manufacturers and some automation suppliers of its effectiveness.
I asked suppliers of automation hardware and software, MES and ERP solutions—and system integrators—where they are on their AI journey and what issues are holding them back. I also asked them to list their three most important problem areas out of the ten that Chat GPT named. The results are in the sidebar “AI Issues in Descending Order As Cited by Interviewees.” I then asked what they felt was holding back food manufacturers from investing in AI tools, and a summation of the results can be found in the box, “What’s Holding Back Investments from Food Manufacturers in AI.”1
Image courtesy of Getty Images / Sarawuth 702
by Wayne Labs, Senior Contributing Technical Editor
AI Issues in Descending Order of Importance As Cited By Interviewees
1. Model complexity
2. Insufficient or inaccurate data
3. Lack of context
4. Uncertainty and noise
5. Human-computer interaction
6. Changes in operating conditions
7.* Inadequate training
7.* Adversarial attacks (manipulating inputs to produce incorrect decisions)
7.* Bias in data
7.* Model drift
*Tied with same number of responses, least important
The Road to AI: Creating New AI tools for Manufacturing
“As a comprehensive advisory firm, we provide digital solutions—including AI-integrated platforms like Salesforce, Microsoft D365 and NetSuite—to foster organizational transformation,” says Kate Brown, senior manager, Wipfli LLP, a Control System Integrators Association (CSIA) partner member. “These products are now embedding AI capabilities directly into their solutions. We recommend that manufacturers start [their AI journey] by leveraging the AI capabilities available within their existing tech stacks and then making incremental improvements.”
For large automation suppliers like Rockwell Automation, expect to see AI tools built into most all major applications, and ones that don’t have embedded AI, soon will. According to Richard Resseguie, product manager analytics, FactoryTalk Analytics, LogixAI, FactoryTalk Smart Object and FactoryTalk Analytics already have embedded AI, and GuardianAI will be available early this year.
Autoware, an automation supplier and CSIA certified member based in Italy, expects to incorporate AI into its software the first half of this year but has been taking a studied approach to implementing AI. “Our main focus is MES, and so we are working to augment MES capabilities using AI,” says CEO Luigi De Bernardini. “Our strategy is to combine process data provided by PLCs and SCADA with transactional data managed by MES and use AI to augment the operator’s expertise in running production. At the moment we are not planning to use AI to close any control loop, but to provide operators the suggestions that can help run the plant smoothly and efficiently.
“We developed the concept of ‘Golden Day,’ deriving it from the concept of a ‘Golden Batch.’ So, a Golden Day in manufacturing is a day in which everything goes as expected. We believe AI can be a tremendous tool to help operators to make each day a Golden Day,” adds Bernardini. Autoware’s first applications of AI include support for root cause analysis (for line stoppages) and an executive summary of production reports.
“Festo offers a suite of software that enables food and beverage processors to improve their OEE and reduce their energy usage by using AI for predictive maintenance, quality and energy savings,” says Lea Bayer, digital sales expert. The Festo AX suite includes software to access data, contextualizing and modeling it. The machine learning (ML)/AI algorithms can detect anomalies and predict errors before they become an issue.
With respect to AI, Gray Solutions’ focus has been on advanced vision systems—mostly in the area of quality inspections, says Gregory Powers, VP of cool stuff. “Visual inspection automation (VIA) software goes beyond the capabilities of traditional machine vision in detecting anomalies and defects, even when products have natural variations. Using proven vision AI technology, manufacturers can scale production, reduce waste and adapt to workforce changes while achieving even higher levels of quality control.”
Gray Solutions, a CSIA member, is exploring embedding AI to make a smart HMI. “Instead of having operators control the process, using AI we can assist—and in some cases control—the process,” says Powers.
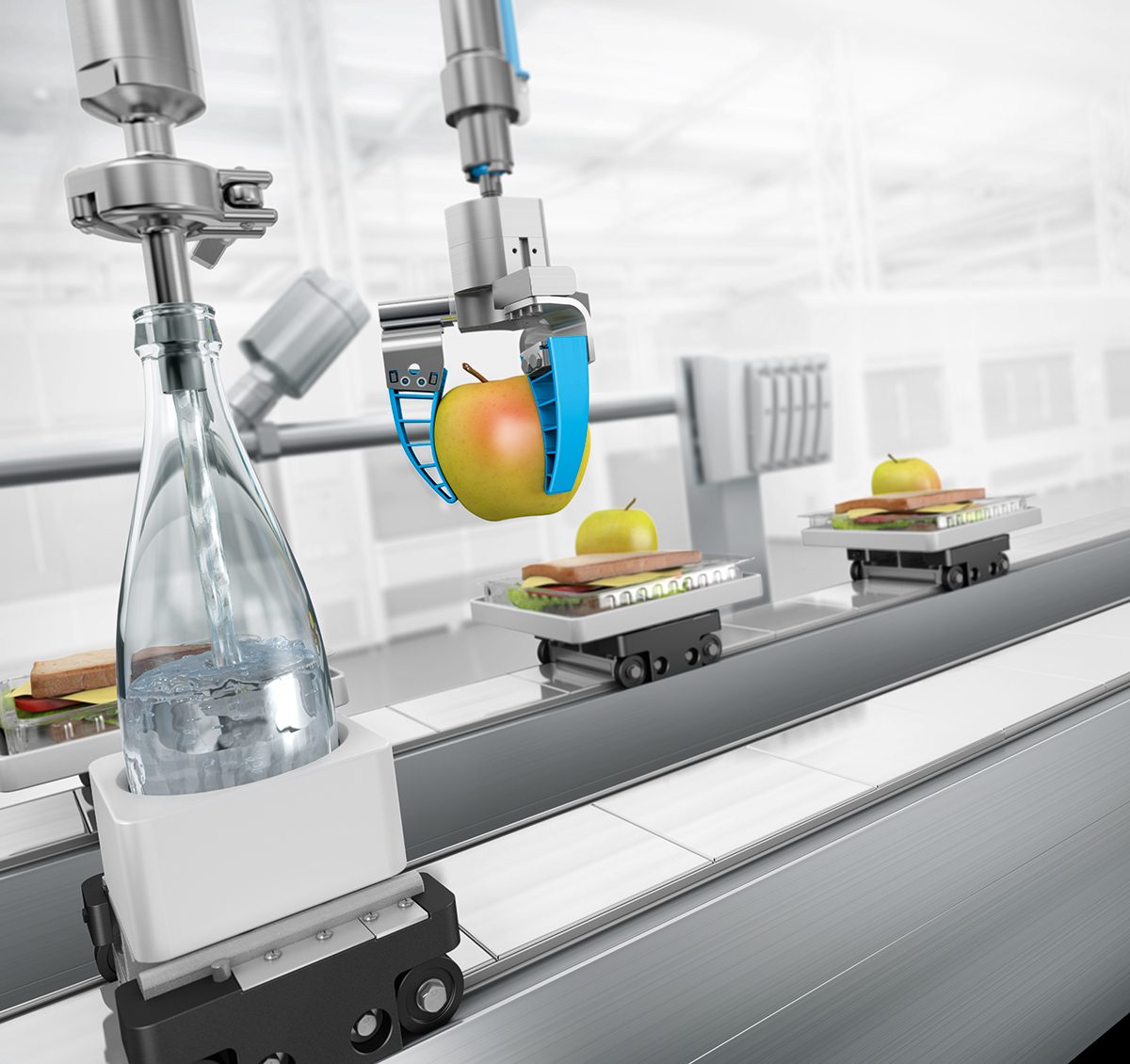
AI powered robotics can make decisions on the fly and adjustments during operation if necessary. When the system makes errors, it can learn from them to speed up operation without stoppages. The system can also alert operators ahead of time when maintenance is due to prevent stoppages. Image courtesy of Festo
What’s Holding Back Investments from Food Manufacturers in AI
According to a recent McKinsey report, large companies globally have captured, on average, only 31 % of expected revenue lift and 25% of expected cost savings from their digital and AI transformations.1
There are several reasons that food manufacturers are waiting to implement AI projects.
• Lack of on-hand historical data to create context in a complex project
• Lack of clarity on what AI is
• Food processors need proof of concept—their perception is that implementation cost is high, and there will be a lack of benefits
• Budget, scope creep
• Risk aversion
• Limited human resources to dedicate to an AI project
• Inadequate training
• Adapting to change that AI may impose
• Unpredictability of AI systems
• Regulatory compliance
• Data security
• Selecting competent vendors and engineers
• Lack of proper IT investment
ERP software providers are embedding AI as well. Infor has a multi-prong approach to leveraging AI/ML across its enterprise portfolio, says Sandeep Anand, senior director, augmented intelligence solutions. “The concept of our embedding strategy applies to three main categories: in-context AI-based recommendations while performing regular operations within business applications, AI-based process automations based on repetitive business actions, and lastly, AI-based predictive and prescriptive analytics to drive operational excellence.” A fourth category deals with using AI-based “conversational” capabilities to augment customer experiences.
Pinnacle offers an AI-based solution called Newton, says Brad Moore, principal, reliability. The system is designed to collect facility data (including live operating parameters from the production line), work order history from the computerized maintenance management system (CMMS), predictive maintenance data (e.g., vibration readings, oil analysis results, thermography readings) and other data used by the system to manage the process. Newton applies this data to calculate near-real-time probability of failure and utilizes a system model to determine the consequences of failure. This risk calculation is evaluated by the AI engine to determine the expected failure date and recommend mitigating tasks to help prevent that failure or delay the expected failure date.
According to Pinnacle’s Rayce Morgante, lead, AI is used in the company’s automated document processing and contextualization. “Using AI, we can ingest large amounts of unstructured data (documents, pictures, emails, etc.) and automatically organize it all in a way that makes it usable for operations and reliability. Once documents are organized, we can extract data into tabular format so that it can be stored in a relational database for later use.”
RoviSys, a CSIA certified member, is pioneering the use of Autonomous AI (see sidebar, “What is Autonomous AI?”) for manufacturing users, including food and beverage processors, says Bryan DeBois, director, industrial AI. Autonomous AI was developed within Microsoft, and one of its first success stories was working with PepsiCo to make more consistent Cheetos.2 Microsoft has since moved away from Autonomous AI to focus its efforts on generative AI. However, RoviSys has continued to invest in the approach, and has brought it to even more manufacturers.
What is Autonomous AI?
Autonomous AI came out of Microsoft, and the concepts are outlined in the O’Reilly book, "Designing Autonomous AI," by Kence Anderson. It is an AI methodology that encompasses four primary concepts:
1: Deep Reinforcement Learning (DRL) – a novel machine learning (ML) algorithm that was created by Deep Mind, a Google spinoff. It was originally demonstrated to reach super-human capability in playing games such as Go, chess and StarCraft.
2: Machine Teaching – capturing subject matter expertise into a graph of concepts, skills and heuristics that the DRL uses to learn better and faster.
3: Simulation – unlike other ML algorithms, DRL learns by doing—not by data. So, it requires a simulation of the part of the process we are trying to optimize. That can be a first principles simulation (based on physics, etc.), but we can also develop a simulation based on historical data or other available equations.
4: Incorporating existing control and optimization strategies – Autonomous AI is not limited to using only DRL and can incorporate (via the machine teaching step) other established control and optimization strategies.
—Bryan DeBois, Director, Industrial AI, RoviSys
Dealing with AI’s Problem Issues
The two most important objectives for manufacturing facilities, especially food and pharmaceutical processing, are reliability and regulatory compliance, says John Keefe, Pinnacle senior engineer. Improving reliability at all levels of the organization is the most value-adding objective so the top three problems for AI to solve would be model complexity, lack of context and adversarial attacks.
“In general, regulators don’t care if a manufacturing facility is reliable, so manufacturers cannot focus only on improving reliability,” says Keefe. “In my own experience with ChatGPT, I found it did not fully understand regulations, so the response to one of my questions was erroneous. This is an example of why lack of context and human-computer interaction are two problems to solve that would prevent wrong decisions about regulatory compliance.”
“From my perspective, the three biggest problems of the ten are insufficient or inaccurate data, lack of context and model complexity,” says Ryan Thompson, CRB senior specialist, industry 4.0. “Insufficient data and a lack of context are related issues, and the vast majority of food plants I’ve seen suffer from this. With respect to complicated models, this is often related to a staffing issue, as food manufacturers often lack the data scientists needed to work within these models.”
“CRB is a Seeq partner, which supports machine learning models,” adds Thompson. “Leveraging a partner here is a great way to begin contextualizing data and building machine-learning models even when you don’t have the trained in-house staff to get you there.”
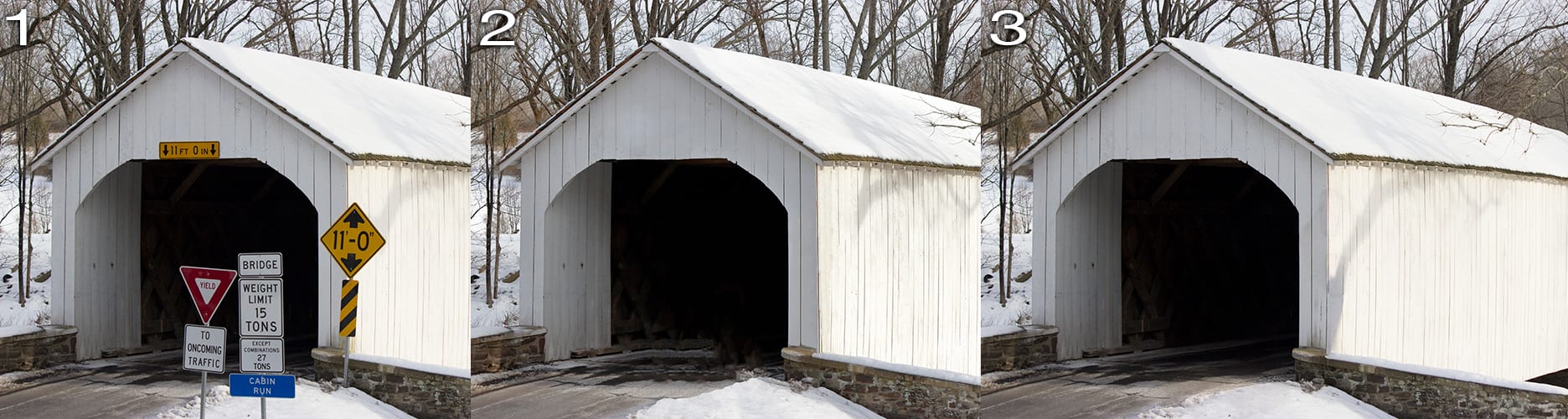
AI certainly has played a role in vision systems and will continue to get better, but it also can save time in image editing applications. In this series, (1) a photo of a Bucks County, Pa., covered bridge has ugly road signs in front of it. (2) The second image shows the signs removed manually with the help of Photoshop, an arduous task taking a couple of hours to complete. (3) The third image shows the signs removed in five steps—taking a total of less than 15 minutes—with the help of Luminar Neo generative AI technology. Photos courtesy of Wayne Labs
“Lack of context,” says Dustin Johnson, Seeq chief technology officer. “When it comes to generative AI, models are usually trained using public datasets that represent common human knowledge and are available on the internet, but they lack private knowledge. Because it is difficult to remove inherent biases present in training data, this can potentially render some results inaccurate. Furthermore, training models with domain-specific private data is both onerous and technically difficult.”
“Data without context is like a jigsaw puzzle with missing pieces; it’s meaningless and can’t be used to form meaningful insights,” says Rockwell’s Resseguie. “Enriching IT data with operational technology (OT) data and vice versa, provides businesses with a comprehensive view of their operations and enables leaders to make data-driven decisions. Lack of context can also drive spurious relationships, since AI without context can be particularly susceptible to finding correlation without causation.”
Johnson adds insufficient or inaccurate data to the list. “Before an organization can begin augmenting data analysis of systems with AI, it must assess its data quality. Accessible, high-quality data is essential for AI effectiveness, as well as relevance of the data for the specific problems being solved and the teams working on them.”
Unfortunately, most plants have collected data for quite a long time, but the data are not organized for the sake of AI implementation, says Autoware’s De Bernardini. “There’s no guarantee that any needed data are available, correct and well maintained. So, data availability and quality are the most important problems to solve.” Collected data and repositories must be set up with AI in mind from the beginning.
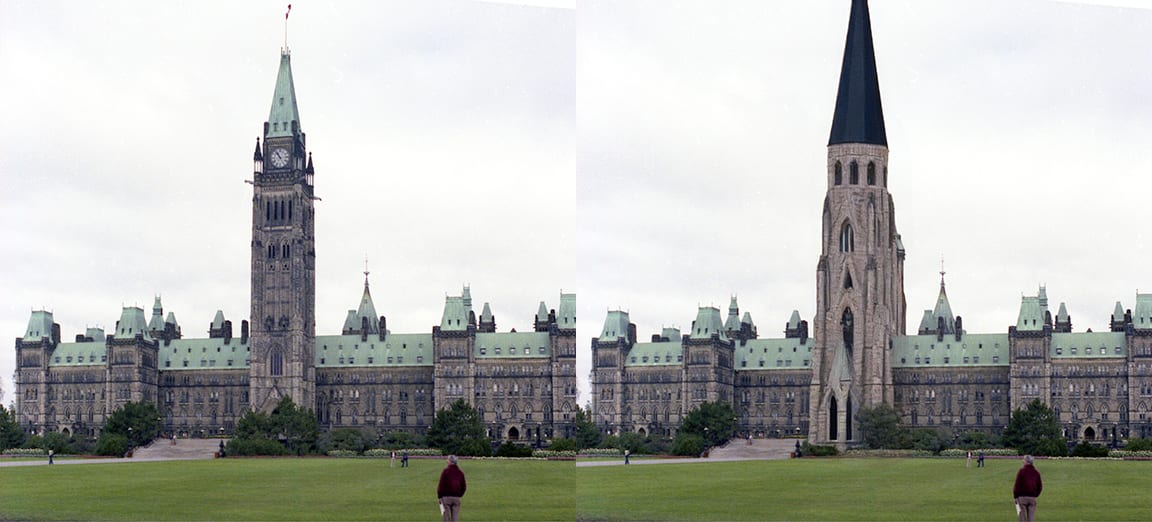
In this second series of images, (1) a straight shot of the national Parliament building in Ottawa, Ont., had its clock tower replaced by (2) a “church steeple” using Luminar Neo AI replacement. This generative AI technology is still in its infancy, uses cloud technology and can result in a good or totally whacky image. Photos courtesy of Wayne Labs
AI and People
And, don’t forget human-computer interaction, says Johnson. Despite popular discourse, AI requires human oversight to function effectively. It does not replace the need for domain experts, but instead it complements their expertise.
This may be easier said than done. Festo’s Boyer listed insufficient or inaccurate data, model complexity and lack of context as three key issues with AI, “but the most important problem is the lack of plant personnel resources to partner with suppliers on the implementation of AI.”
The people aspect affects the adoption of AI into the manufacturing environment. Gray’s Powers says, “Tribal knowledge of how processes work is engrained in the operators, and introducing something else like AI tends to be a distraction. Now, this is even more reason to adopt AI. This tribal knowledge can be the key to teaching AI how to solve problems.”
“The human factor is the most unpredictable one in a factory,” says Autoware’s De Bernardini. “For this reason, all the processes that have a human contribution face changes in the operating conditions. If correctly collected, this information can be used to feed AI models and generate recommendations. If not, they introduce a level of unpredictability that can compromise the success of the developed models.”
“Human-computer Interaction is my best pick from the list, because having actionable, transparent models that provide end users a way to explain recommendations and understand the strengths and weakness of the predictions is important and essential,” says Infor’s Anand. “I would say that some of the others in the list are driven from the perspective of an AI practitioner and not necessarily an end user, but they all point to the issue of an AI model giving wrong results.”
Model Complexity
“Uncertainty and model complexity are the areas in which manufacturers have the most value to gain,” says Pinnacle’s Morgante. “Often the complexity of a manufacturing facility is anecdotally understood, and the uncertainty it produces is apparent. But there is often a failure to incorporate these concepts into models used around the facility. In fact, uncertainty is rarely quantified specifically, and it is one of the most important factors in how a facility’s reliability of operations should be managed.”
“Model complexity can lead to wrong decisions for improving reliability because unreliability has a wide variety of causes including physical equipment failure, human error and poorly designed manufacturing processes,” says John Keefe, Pinnacle senior engineer.
Since the manufacturing operations are a continuous evolving system, the models need to be adapted continuously, says De Bernardini. “Models very complex to build will be extremely complex to maintain. For this reason, we are looking at a self-tuning model that can evolve as the operations evolve.”
To Powers, model complexity has always been a key issue in adopting AI. “We at Gray Solutions have been using models/simulation software for the past four years. In this modeling, we perform experiments within process areas to determine results or best outcomes. Now when we introduced the same process using AI algorithms, we found different results. The reason for this is that with AI we introduce much more complexity with too many variables to consider. We then have to redefine the AI algorithm to focus on the variables that have the biggest impact on the model.”
Changes in operating conditions, uncertainty and noise, and model complexity are the most important issues to address, contends RoviSys’ DeBois. “These are fundamental realities of life on the plant floor and are almost completely ignored by traditional AI approaches.” Des Bois believes that Autonomous AI, which includes deep reinforcement learning (DRL), is better suited to handling these issues. DRL is known for being able to make optimal decisions, even in the face of novel situations. “In the case of noise, we actually do not want perfect simulations, and will introduce artificial noise to replicate the types of sensor issues that are common on the plant floor. And model complexity is addressed through our use of machine teaching, which breaks a monolithic model down into simple decision-making activities.”
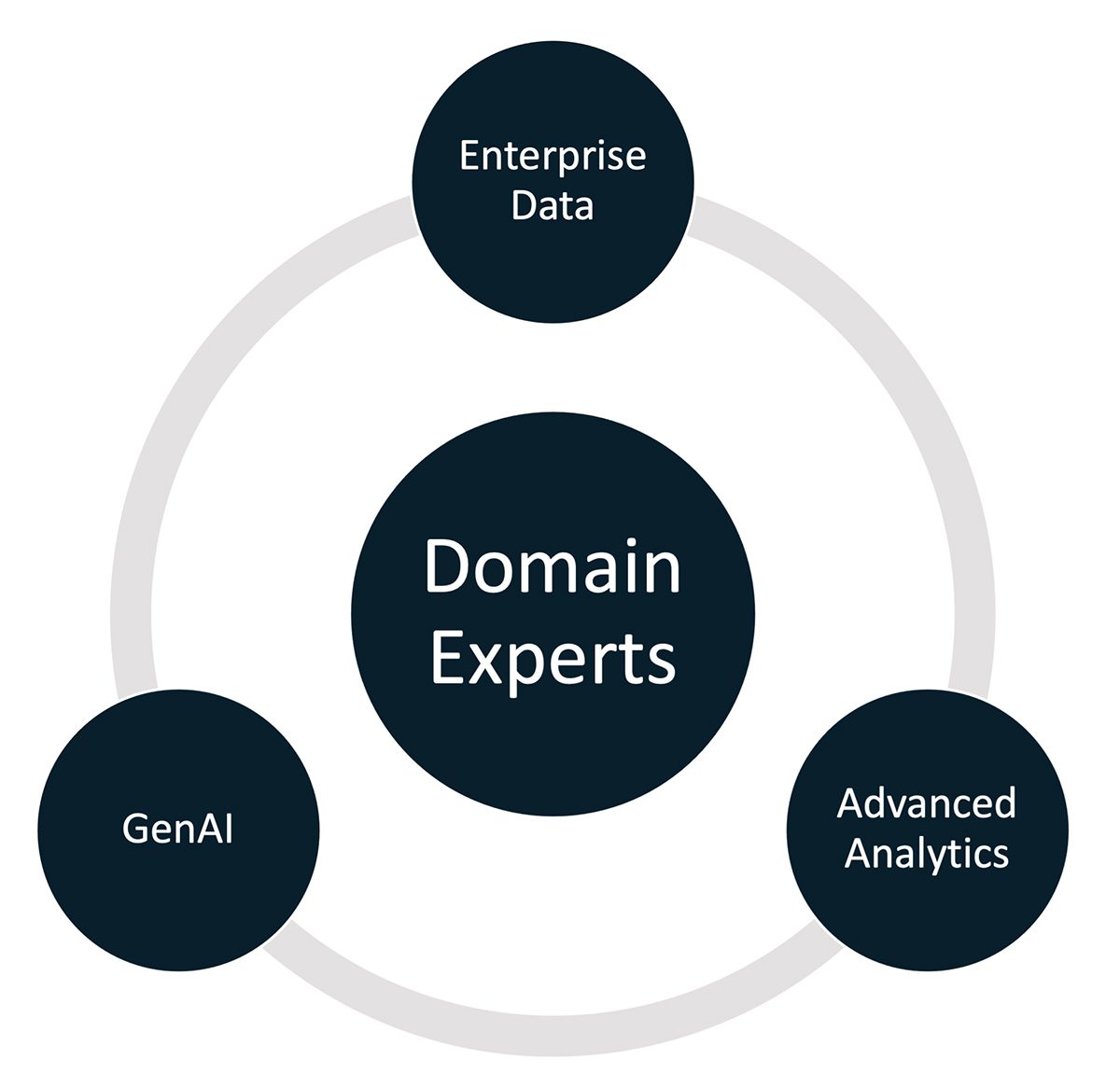
Achieving manufacturing success using AI requires empowering domain experts with the key ingredients—reliable enterprise data, advanced analytics, and GenAI—to analyze information efficiently and make effective operational and business decisions. Image courtesy of Seeq
To deliver reliable and efficient control systems in demanding industrial environments, industrial control suppliers must effectively handle uncertainty and noise in data, says Rockwell’s Resseguie. AI assists in gathering data from multiple sources (e.g., sensors, historical records and external databases) to create a comprehensive and accurate picture of the system being monitored. Collecting data simultaneously helps reduce errors, or “noise,” in the data, making it easier to use in information system applications without having to clean it up first. Integrating AI-based solutions into data processing pipelines can reduce data noise significantly, leading to improved data quality, better decision-making and enhanced overall system performance.
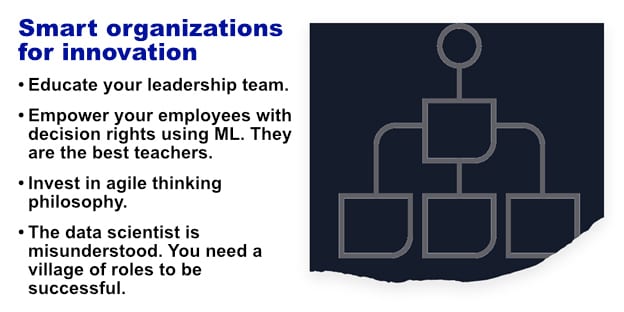
With the continued focus on AI, this is a good time to invest and grow organizations to leverage technology successfully. This can begin as a top-down approach first, but should reach out to employees to give them to tools they need to make good decisions. Image courtesy of Infor
Teamwork Counts on AI Projects
When asked how suppliers and integrators are working with food processors on AI projects, the overwhelming response is as a team. “You have to walk before you can run,” says CRB’s Thompson. “I am working primarily with our food manufacturing clients on structuring and collecting data and focusing on IT/OT integrations. Most of these clients are not mature enough in the data space to begin working with and taking advantage of AI. As a services provider, our offerings are different for each client, and I believe they will remain that way. OEMs have a tremendous opportunity to build AI as a service offering into their machines to leverage their network and equipment expertise to deliver unique offerings.”
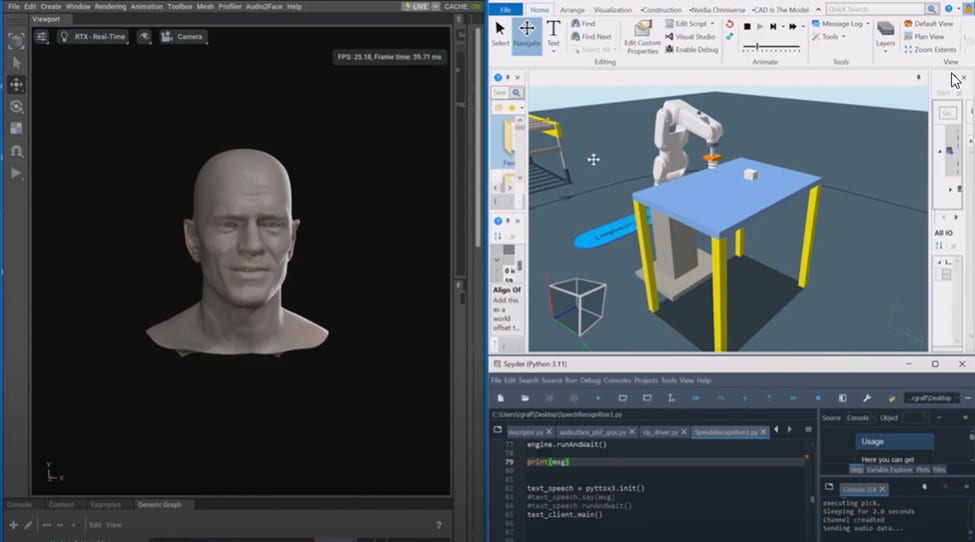
Integration of ChatGPT with an avatar to control machinery shows an AI approach that system integrators have been researching to create practical AI-driven systems. Image courtesy of Gray Solutions
“We are always working as a team with our customers,” says Festo’s Bayer. “We have regular meetings and open channels of communication, and are all committed to the same goal. We concentrate on standard solutions in terms of technical integration and the assets that Festo AX is analyzing. While there is always a need for individualization, the lack of end user human resources points to standardization as a more efficient way of gaining results.”
“This article is very appropriate because it discusses the general challenge with AI and any advanced analytics in the food and beverage industry,” says Infor’s Anand. “For the highly specialized solutions that require multiple ML models, different groups working together and highly personalized space like manufacturing (where every product has the propensity to be different based on materials used), we acknowledge the need for people and technology to work together continuously. We call this augmented intelligence.”
“Most of our customers are running at full speed all the time, so shutdowns aren’t an option to try new things,” says Rockwell’s Resseguie. “Our approach involves connecting to the data their equipment generates already and using products which accelerate machine learning near the equipment. The prescriptive outputs from these processes have a human in the loop to take action so operators and management can both ease into the changes that AI brings.”
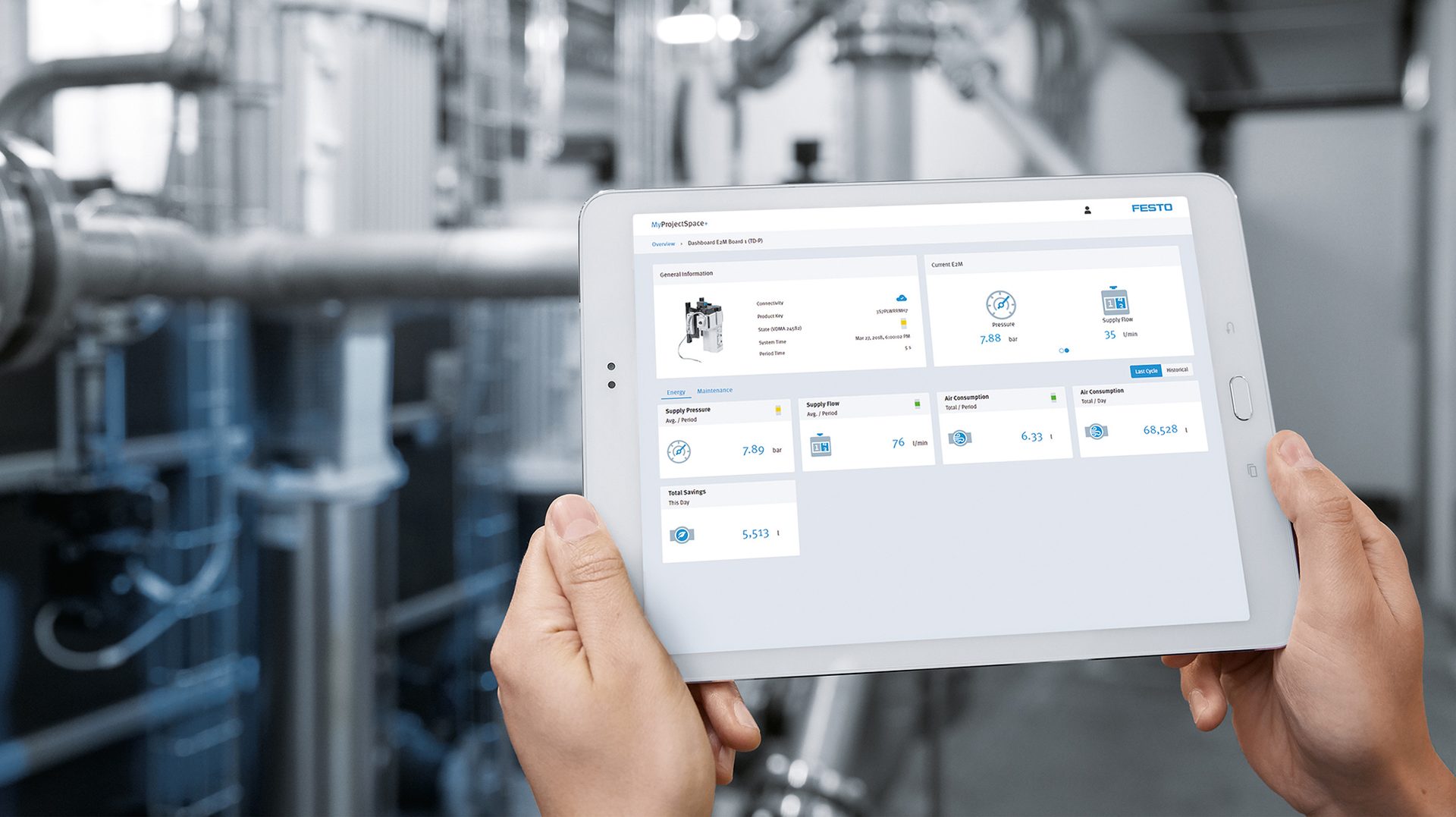
Festo dashboard shot of predictive analytics for an air prep system uses the Festo AX suite, which includes software to access data, contextualizing it and modeling it. The AI/ML algorithms can detect anomalies and predict errors before they become an issue. Image courtesy of Festo
“To solve the problems of our food processing clients, they have built cross-functional teams that work closely with our team to tackle these complex issues,” says DeBois. “The solutions to these problems are as varied as the problems themselves, so RoviSys is never looking to build a tool for an entire client base, but instead develop a solution that is tailored directly to the customer’s needs.”
Finally, embedding AI into applications such that users don’t have to deal with AI directly makes it simpler to apply and requires fewer people and less time to implement. “Simplicity is key,” says Maggie Slowik, IFS global industry director for manufacturing. “The last thing we want is for our customers to go and buy a ‘bag of AI’ or ‘AI black box.’” IFS recently launched its IFS.ai ERP suite, an IT architecture whose AI capabilities are woven into all of IFS’s products and industry-specific capabilities, making AI widely available across a manufacturer’s organizations. Food processors can simulate, optimize and automate processes directly related to their needs—and across the manufacturer’s many sites and related businesses. FE
References/Resources
[1] “Rewired for Value: Digital and AI Transformations that Work,” McKinsey Digital, July 31, 2023
[2] “More Perfect Cheetos: How PepsiCo is Using Microsoft’s Project Bonsai to Raise the (Snack) Bar;” Leah Culler; Dec. 17, 2020; Microsoft — AI Blog for Business & Tech
“AI: To Be Seen and Not Heard,” FE, June 2023
“AI: Behind-the-Scene Partner in Process Applications,” FE, June 2023
“AI/ML-based Real-time Sensing Techniques Turn Art of Winemaking Into Exact Science,” Engineering R&D, FE, July 2022
“AI in Process Control,” FE, June, 2022
“AI Helps Control Greenhouse Automation Systems,” FE, Engineering R&D, June 2022