Automation
Which kind of productivity tool or platform is needed as companies take on continuous improvement projects, smart manufacturing upgrades or pilot programs?
Calls for New Productivity Tools
Increased Throughput
A large brewer with advanced plant floor technology lost $50 million in one year due to unplanned downtime, according to Brad Moore, lead principal of reliability at Pinnacle. The financial pain is real for food manufacturers as increased volume puts pressure on operations to find strategies to understand inefficiencies within production data better.
Not surprisingly, companies are ramping up continuous improvement initiatives, including overall equipment effectiveness (OEE), throughput, capacity utilization, key performance indicators (KPI), unscheduled downtime, overall operator effectiveness, and many other metrics. Food brands are evaluating new data productivity tools to achieve efficiencies—and many choices exist.
Image courtesy of Getty Images / Gerenme
by Grant Gerke
A Growth Mindset and Selecting Productivity Tools
There are many plant floor optimization challenges in 2024. However, adding data management tools is especially difficult with workforce challenges, increased product throughput and line data, and the transition from analog to digital.
Many companies are finding smart manufacturing pilot programs and one-off digital initiatives have their positives and negatives. Some industry analysts advocate for long-term approaches. “(Companies) need to avoid isolated digitalization use cases since you might not get the return on investment that companies need,” says Line Egelund Pederson, partner at Emendo Improvement, in a recent webinar. “Companies must invest in the digital tools that allow your company to mature.”
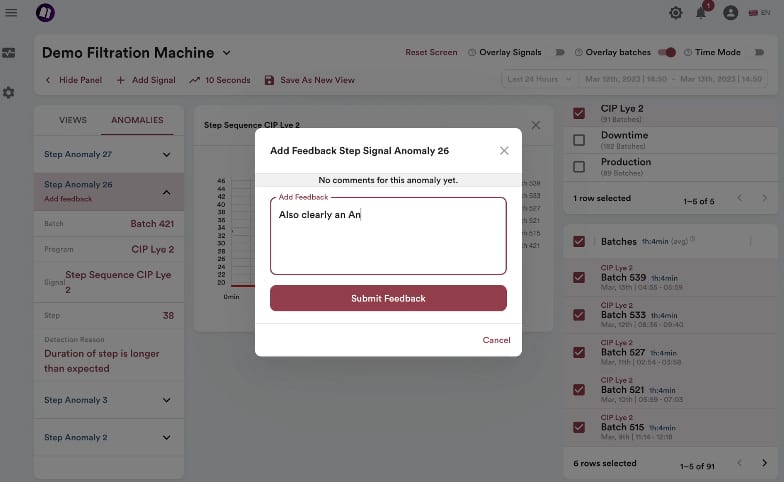
The AI platform gives dairy operators a natural process to interact with the technology. An operator can ask for a root cause analysis, solve the issue and provide feedback for the system through natural language conversation. Image courtesy of MontBlancAI
However, getting alignment for data management objectives, continuous improvement, and current production demand is difficult for large and small food manufacturers. Data management technology is moving so fast that companies are moving to rapid improvement projects or experiments, according to Lisa Zasada, director of reliability and improvement at General Mills. Zasada defines General Mills’ experiments as being much smaller than a pilot project and a new way of operating, solving a problem or (implementing) a new technology.
“I try to avoid the word pilot and move further to proof of technology and of value as very clear terminology because it points to an endpoint by which we're going to make a decision as an organization,” Zasada says. “Understanding what you're trying to drive toward to add business value allows flexibility if a proof of technology or value doesn't go the way you expected.”
“The term Industry 4.0 and Digital Transformation encompasses just too much,” says Markus Guerster, founder and CEO of MontBlancAI. “If you now add AI into the mix, nobody actually knows anymore what it means to have AI-enabled Industry 4.0 in a factory—it’s just a couple of meaningless buzzwords. What brought immense ROI for some, doesn’t work for others.”
"To train AI, many platforms need upfront data labeling. Humans need to tell the system for every set of data what is good and bad. Imagine doing this for just one cleaning machine, which cleans over a hundred objects and collects hundreds of measurements. It doesn't scale.” — Markus Guerster, Founder and CEO, MontBlancAI
Guerster says the approach at MontBlancAI is based on fast, agile iterations. “Instead of throwing around buzzwords, we focus on action. Instead of setting up a million-dollar project with milestones defined for the next few years, we work with our customers to identify low-hanging fruits that we can solve in two weeks,” says Guerster. “The initial first steps are primarily about learning. It’s a constant cycle of problem identification, implementing a solution, collecting feedback and repeating it.
The MontBlancAI software platform offers experimentation without the need for an internal team of expensive AI experts. The platform leverages unsupervised learning of production processes without the need for time-consuming data labeling.
“To train AI, many platforms need upfront data labeling. Humans need to tell the system for every set of data what is good and bad,” says Guerster. “Imagine doing this for just one cleaning machine, which cleans over a hundred objects and collects hundreds of measurements. It doesn't scale.”
Recently, a leading German dairy turned to MontBlancAI technology to continuously monitor and validate its clean-in-place (CIP) processes. The dairy sources milk from more than 2,000 farmers and processes more than two million liters per day into a variety of dairy products.
The process starts with MontBlancAI’s proprietary data pipeline and connects to the dairy’s CIP’s programmable logic control (PLC)—a Siemens S7 PLC—via an OPC-UA industrial networking interface. Once connected, the system starts with unsupervised learning of data points and begins understanding what normal looks like. For example, the platform learns the range of a typical temperature profile if a tank is cleaned with lye.
“Once our system is connected, it collects data, visualizes it and automatically learns what a golden batch looks like,” says Guerster.
From there, the platform moves to anomaly detection. The process compares new data points with the learned temperature range. If out of range, the AI platform triggers alarms and escalates the issue. The issue can be escalated via emails, phone calls, text messages, Microsoft Team notifications or internal notification systems.
To solve and correct the errors, the AI platform gives dairy operators a natural process to interact with the technology. An operator can ask for a root cause analysis, solve the issue and provide feedback for the system through natural language conversation.
“There's no big form to be filled out, it’s done through natural conversations,” says Guerster. “We use generative AI to classify that data, helping the user have conversations, and feed it back into our system. At this point, the system starts data labeling to provide qualitative feedback."
More than 5,000 cleanings have now been validated with various improvement areas being identified that allow the dairy to reduce its cleaning time by hours and save thousands of cubic meters of freshwater, acid and lye, according to the customer.
“We feel our system is unique since we turn data into actionable insights,” says Guerster.
Smaller Food Manufacturers, Big Decisions
Small food brands are also moving fast to stay current and are using data workflow tools to meet various goals, including product customization demand and Food Safety Modernization Act (FSMA) requirements. Recently, Companion Baking Company, a small wholesale bread producer based in St. Louis, moved away from manual documentation and chose a workflow platform that streamlined its processes for its 320 SKUs.
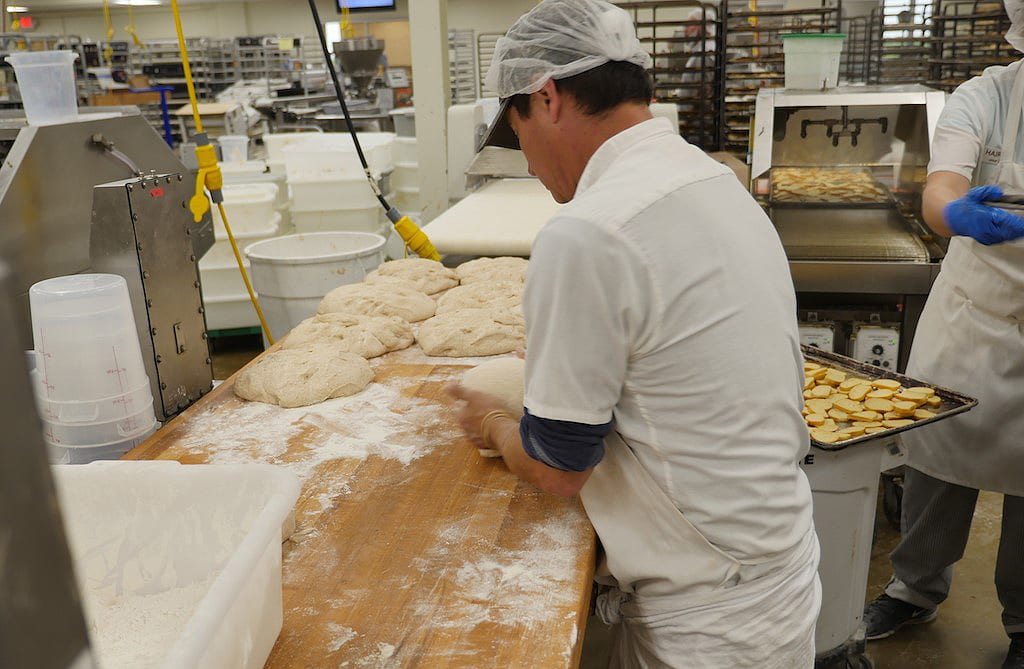
Companion Baking Company, a wholesale company, needed a workflow platform for its operations so bakers could communicate, track and input data for its 320 SKUs. Image courtesy of QAD Redzone
To do this, Companion Baking chose QAD Redzone’s productivity platform. QAD Redzone’s Connected Workforce Solution offers four components—productivity, compliance, reliability and learning—and the wholesale company added the productivity module first before rolling out the other three components. QAD Redzone’s platform offers a range of visual communication tools and metrics, including OEE performance, line status, a digital fishbone, action management and collaboration tools, like chats, huddles and daily forums.
The digital transition for the bread company started with its need to understand operational processes at its 45,000-sq.-ft. facility, which employs more than 65 frontline workers. The baking teams use semi-automated processes, including large mixers to make the dough, four bread-dividing lines and one pastry line. The bread and pastries are baked in ten rack ovens and two deck ovens.
According to Suman Shekar, operations manager at Companion Baking, “The company differentiates itself by conducting R&D, customizing products and developing smaller batches to meet customer needs.” With so much product customization at the bakery, Shekar realized the company needed to articulate and streamline its processes before employing Redzone’s digital workflow platform.
Shekar executed time studies across 320 SKUs and optimized activities for those products. “Every baker said it would be nice if we could communicate digitally about the schedule, whether they’re behind, on-time or doing well for the day,” adds Shekar.
With QAD Redzone’s platform, Companion Baking’s first step in its 90-day agile sprint included connecting dividing machines, case packers and other equipment. The next step was to give its frontline workers a digital identity and enable them to communicate, track and input data. QAD Redzone also assisted in acquiring and deploying tablets—monitor line productivity—specifically upgraded to withstand a harsh factory environment.
The Companion Baking team set out to achieve five standard measurable lean journey goals during a 90-day sprint:
● Creating a visual factory (OEE measurements in real-time)
● Coaching on lead production techniques and collaborative problem-solving skills
● Improving OEE by eight points by the of the sprint
● Achieve cost savings
● Sustaining all gains made in the first 90 days
The process had challenges, as employees needed to show more support toward documenting their processes at first. However, increased team buy-in over time accelerated the drive to capture more processes. “OEE uplift went up 30% in just two months, and by six months it grew to 60%,” says Shekar. “Now, on day-to-day runs, it’s at 80% and above.”
With the compliance module, the bread company also conducts monthly walkthroughs of the bakery and performs dough temperature and barcode checks that maintain compliance with FSMA. Companion Baking says, “The team can now perform a mock recall in just 30 minutes, significantly faster than the two hours it took previously.”
Measuring Success with Digital or AI Platforms
Finding operational savings and ROI is a massive part of the discussion with these platforms, but it should not be the determining factor. Use cases vary, but operational learning should be paramount for legacy and new companies. “The focus should be learning because it's a new technology and it has never been cheaper to experiment with AI,” says Guerster. “In the past, companies needed internal teams to conceive and deploy.”
Companies must address production volume and growth ramifications immediately and let ROI take a backseat. In Food Engineering’s 2023 State of Manufacturing Report, 14% of the food manufacturers that reported an increase in volume production said, “their business saw more than a 25% increase in throughput.”
“The language of data, technology, continuous improvement and manufacturing doesn’t always align,” says Zasada. “Sometimes you can end up in conversations where it’s really difficult to decipher what is being said by different players. This is where the role of growth mindset and learning agility is critical.”
As these case studies show, there are many approaches and digital technology solutions for small, medium and large-sized food manufacturers. FE